In an era dominated by technological advancements, the term “artificial intelligence” (AI) has become ubiquitous, influencing industries from healthcare to finance and everything in between. For startup founders, serial entrepreneurs, and corporate executives, understanding the nuances and types of AI is both challenging and imperative. This article aims to unravel the complexities of AI, breaking down the various types and guiding readers to discover solutions that align with their unique enterprise needs.
The Relevance of AI in Modern Business
Once confined to science fiction, AI has evolved into a dynamic and omnipresent force, influencing sectors ranging from healthcare and finance to manufacturing and beyond. Its relevance is in automating tasks and reshaping entire business models. The ability to process vast amounts of data, recognize patterns, and make decisions without human intervention propels AI to the forefront of technological innovation.
As AI reshapes industries at the speed of thought (to borrow Bill Gates’ words), its transformative power rapidly impacts how businesses operate and innovate. Wherever you are on the business spectrum, you’ll agree that intelligent machines are leading a rapid revolution in the emerging enterprise.
If you understand the nuances of artificial intelligence, you’ll embrace the crucial necessity to steer your company into uncharted but potentially rewarding territories of the digital realm.
Navigating Challenges with AI
The journey into the world of AI is challenging. For those at the helm of startups and established corporations, comprehending the various types of AI and their potential applications can be daunting. The rapid pace of technological advancement often leaves decision-makers grappling with the complexities of AI adoption.
Here are three considerations when adopting AI for your business:
Data Privacy and Security Concerns:
AI systems often require access to large volumes of data for training and learning. This data can include sensitive information, and its handling raises concerns about privacy and security. Enterprises must implement robust measures to safeguard data, comply with regulations (such as GDPR or HIPAA), and ensure that AI models do not compromise the confidentiality of sensitive information.
Lack of Skilled Talent:
The field of AI demands specialized skills, and there is a shortage of professionals with expertise in machine learning, deep learning, and related areas. Finding and retaining skilled AI talent is a significant challenge for enterprises. Without the right experts, implementing and maintaining AI systems becomes more difficult, hindering the successful integration of AI technologies into the enterprise.
Lucky for you, at Iterators, we can help design, build, and maintain AI software solutions for both startups and enterprise businesses.
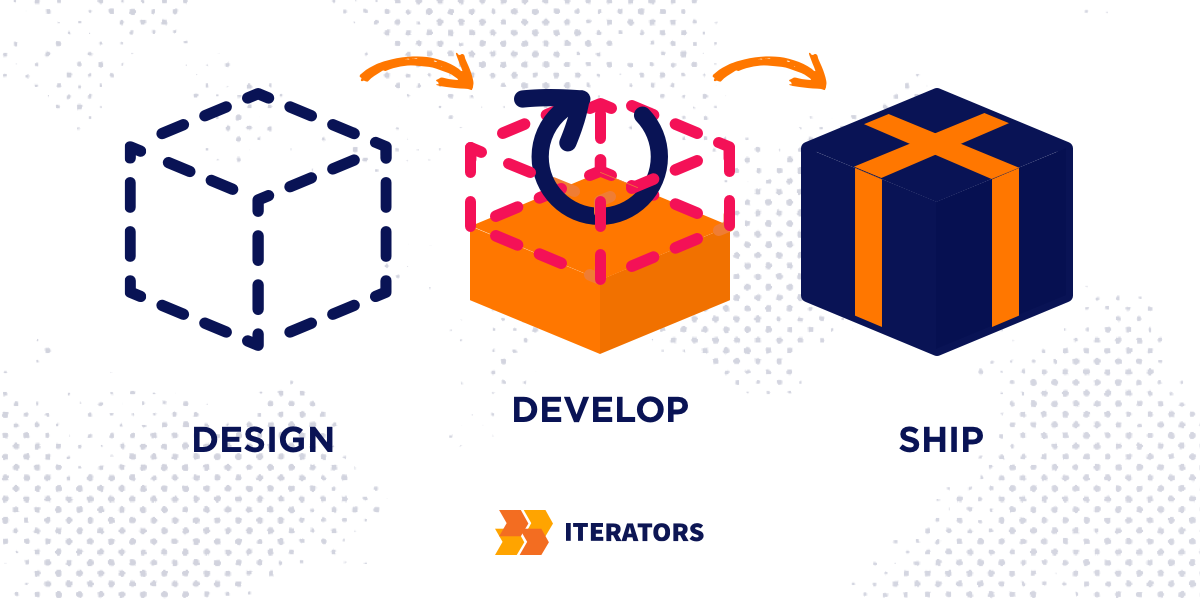
Schedule a free consultation with Iterators today. We’d be happy to help you find the right software solution for your company.
Integration with Existing Systems and Processes:
Many enterprises already have established systems and processes in place, and integrating AI into these existing frameworks can be a complex task. Compatibility issues, interoperability challenges, and the need for seamless integration with legacy systems can slow down the adoption of AI. Enterprises must carefully plan and execute the integration process to avoid disruptions and maximize the benefits of AI technologies.
These challenges highlight the importance of a thoughtful and strategic approach to AI adoption in the enterprise, considering not only the technical aspects but also legal, ethical, and organizational considerations.
Here are three considerations when adopting AI for your business:
- Data Privacy and Security Concerns: AI systems often require access to large volumes of data for training and learning. This data can include sensitive information, and its handling raises concerns about privacy and security. Enterprises must implement robust measures to safeguard data, comply with regulations (such as GDPR or HIPAA), and ensure that AI models do not compromise the confidentiality of sensitive information.
- Lack of Skilled Talent: The field of AI demands specialized skills, and there is a shortage of professionals with expertise in machine learning, deep learning, and related areas. Finding and retaining skilled AI talent is a significant challenge for enterprises. Without the right experts, implementing and maintaining AI systems becomes more difficult, hindering the successful integration of AI technologies into the enterprise.
- Integration with Existing Systems and Processes: Many enterprises already have established systems and processes in place, and integrating AI into these existing frameworks can be a complex task. Compatibility issues, interoperability challenges, and the need for seamless integration with legacy systems can slow down the adoption of AI. Enterprises must carefully plan and execute the integration process to avoid disruptions and maximize the benefits of AI technologies.
These challenges highlight the importance of a thoughtful and strategic approach to AI adoption in the enterprise, considering not only the technical aspects but also legal, ethical, and organizational considerations.
The Imperative to Understanding AI
The imperative to understand AI must be balanced. It goes beyond adopting a buzz-worthy technology; it’s about unlocking unprecedented efficiency, uncovering new opportunities, and staying competitive in a landscape that rewards those who embrace innovation. This article will introduce you to the various types of AI, unique functionalities, and capabilities.
Connecting with AI
Before delving into the technicalities, let’s establish a connection with AI beyond algorithms and data sets. AI is more than just a tool; it’s a catalyst for change, a dynamic partner in the journey towards efficiency and innovation. For startup founders charting the course for their ventures, serial entrepreneurs seeking the next frontier, and corporate executives steering large enterprises, the understanding of AI isn’t just an asset; it’s a strategic imperative.
As we embark on this exploration of the types of AI that work for every enterprise, the goal isn’t just to demystify the technology but to empower decision-makers. From understanding the fundamental types of AI to grasping the emerging trends shaping the future, this article aims to be a comprehensive guide. So, let’s venture into the realm of AI, where possibilities are boundless, and the future is shaped by our choices today.
Understanding Fundamental Types of AI
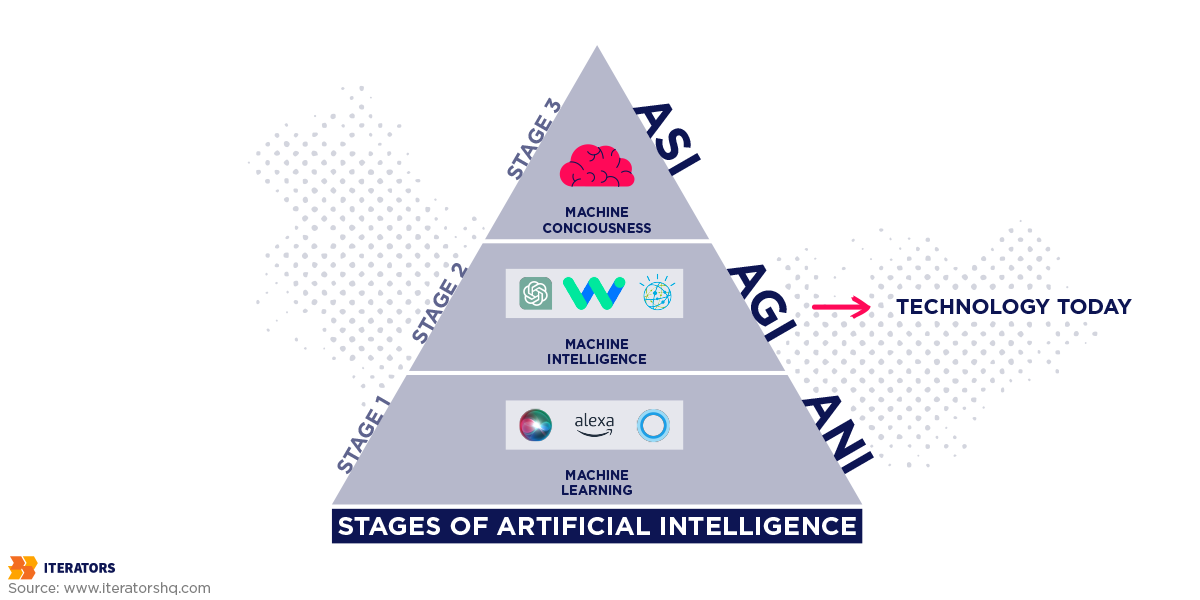
Understanding artificial intelligence begins with comprehending its fundamental types. In this section, we dissect the landscape of AI, distinguishing between Narrow AI and General AI and exploring the nuances of Weak AI versus Strong AI.
Narrow AI vs. General AI
The best way to begin is to grasp the fundamental types of AI: Narrow AI and General AI.
Defining Narrow AI
Also known as Artificial Narrow Intelligence (ANI), Narrow AI epitomizes specialization. It’s bespoke AI tailored for a specific task or domain, excelling in well-defined scenarios. Imagine a voice-activated virtual assistant or a recommendation system that suggests personalized content. These are instances where Narrow AI shines, demonstrating proficiency within the boundaries of its designated function.
The Practical Applications of Narrow AI
There are plenty of practical uses of Narrow AI, from image recognition to language translation and speech recognition systems in smartphones to recommendation algorithms powering streaming platforms. Its focused nature makes Narrow AI an invaluable asset for enterprises seeking precision and efficiency in targeted areas.
The Cognitive Horizon of General AI
In contrast, General AI or Artificial General Intelligence (AGI) can understand, learn, and apply knowledge across diverse domains. It aspires to emulate human cognitive abilities across a wide range of domains.
Unlike Narrow AI, General AI aims for a broader understanding of the world and is capable of learning and adapting to diverse challenges.
The Enigma of General AI
The pursuit of General AI remains an enigma—a theoretical frontier challenging researchers and AI enthusiasts alike. The potential implications of General AI span industries, promising dynamic problem-solving capabilities that could revolutionize fields from scientific research to creative endeavors.
Bridging the Gap with Hybrid AI Models
The synthesis of Narrow AI and General AI births powerful hybrid models. These models combine the depth of specialization seen in Narrow AI with the adaptability and learning capacities characteristic of General AI. A prime example is autonomous vehicles, where image recognition (Narrow AI) collaborates with contextual understanding (General AI) to navigate complex road scenarios.
Successful Hybrid AI Models in Action
Hybrid AI models showcase the connection achievable by blending different fundamental types. The healthcare sector, for instance, leverages hybrid models to interpret medical images with precision while understanding the broader context of patient health, marking a pivotal advancement in diagnostic accuracy.
Understanding the distinctions between these types is paramount for enterprises seeking tailored solutions. For instance, implementing Narrow AI for automating customer support responses or General AI for dynamic problem-solving scenarios can significantly impact operational efficiency.
Weak AI vs. Strong AI
Delving deeper, the dichotomy of Weak AI and Strong AI unfolds.
Weak AI, also known as Artificial Narrow Intelligence (ANI), is designed for a particular task and lacks the broad cognitive abilities of humans. On the contrary, Strong AI, or Artificial General Intelligence (AGI), possesses human-like cognitive abilities, enabling it to comprehend, learn, and apply knowledge across various domains.
Where Weak AI Thrives
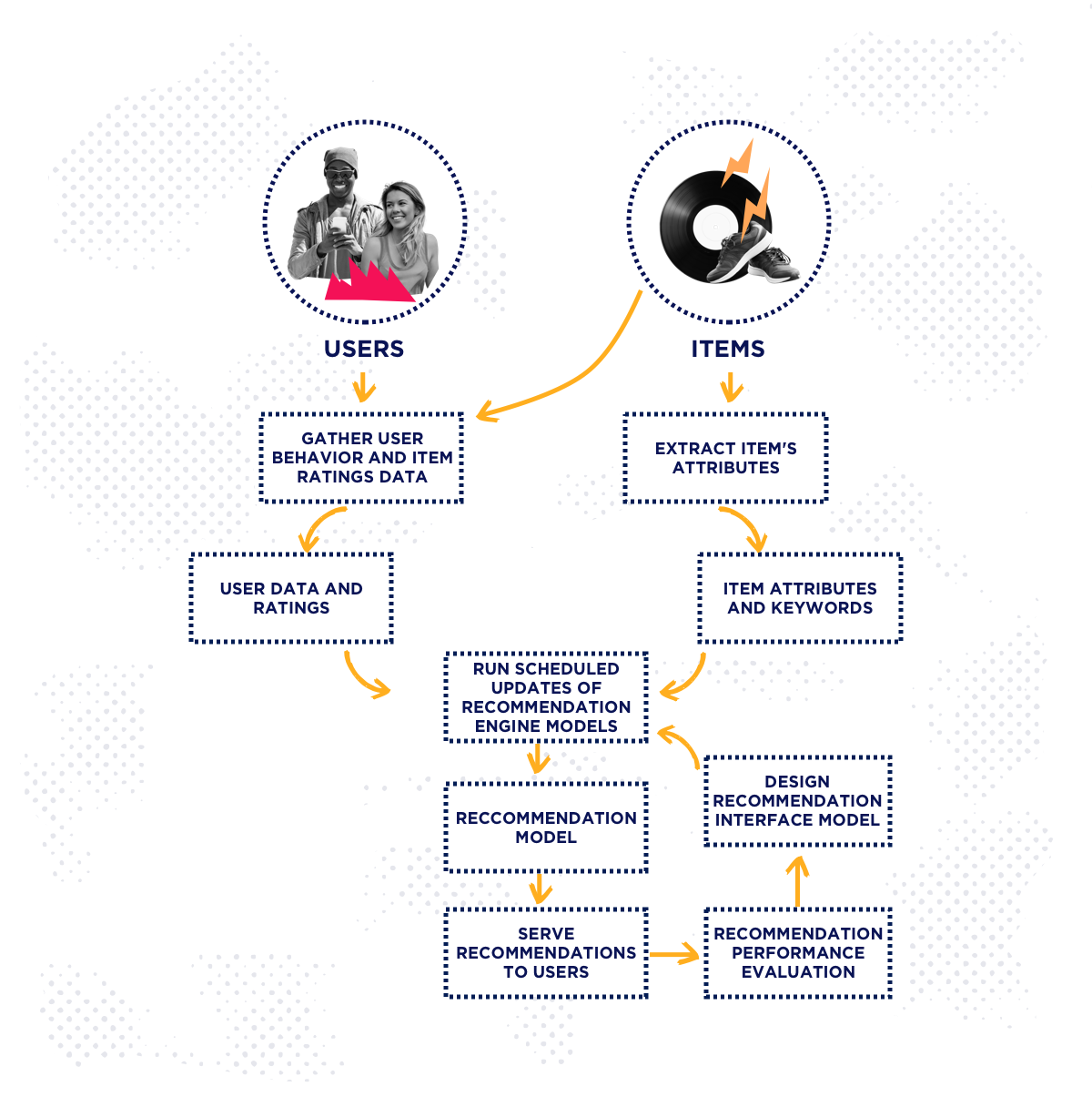
Weak AI thrives in applications where task-specific mimicry is sufficient, providing practical solutions for tasks such as language translation or routine customer service interactions. The key lies in recognizing its limitations—precisely, the inability to transcend predefined functionalities.
Weak AI finds its niche in applications like virtual personal assistants, chatbots, language translation services, and recommendation systems, enhancing user experiences in targeted areas.
Strong AI and the Pursuit of Autonomy
In contrast, Strong AI, or Artificial General Intelligence (AGI), aspires to attain autonomy and comprehension comparable to human intelligence across diverse domains. It encompasses the ability to understand, learn, and apply knowledge flexibly—an ambitious goal that remains largely theoretical.
Contributions of Strong AI to Complex Problem-Solving
While Strong AI has yet to materialize fully, its theoretical contributions to complex problem-solving are profound. From medical research requiring dynamic adaptation to creative endeavors demanding versatile thinking, Strong AI represents a vision of machines capable of understanding and learning across the breadth of human knowledge.
Meanwhile, the theoretical underpinnings of Strong AI prompt researchers to envision solutions for complex problem-solving, with implications spanning from healthcare diagnostics to advanced scientific research.
Hybrid AI as a Synergistic Approach
Once again, the hybridization of Weak AI and Strong AI models emerges as a pragmatic approach. Hybrid AI combines the efficiency of Weak AI’s task-specific mimicry with the potential for broader comprehension and learning seen in Strong AI. This synergistic approach maximizes practical applications while acknowledging the current limitations in achieving full autonomy.
Successful Integration of Weak and Strong AI
Enterprises navigating the AI landscape witness successful integrations, such as language translation services that utilize Weak AI for precise translations and Strong AI elements for contextual understanding, enriching user experiences.
Examples of Successful Hybrid AI Models
The marriage of Narrow AI and General AI often gives rise to powerful hybrid models. Consider the synthesis of image recognition (Narrow AI) with contextual understanding (General AI) in autonomous vehicles. This fusion enables vehicles to identify objects and comprehend their context, a crucial factor for safe navigation.
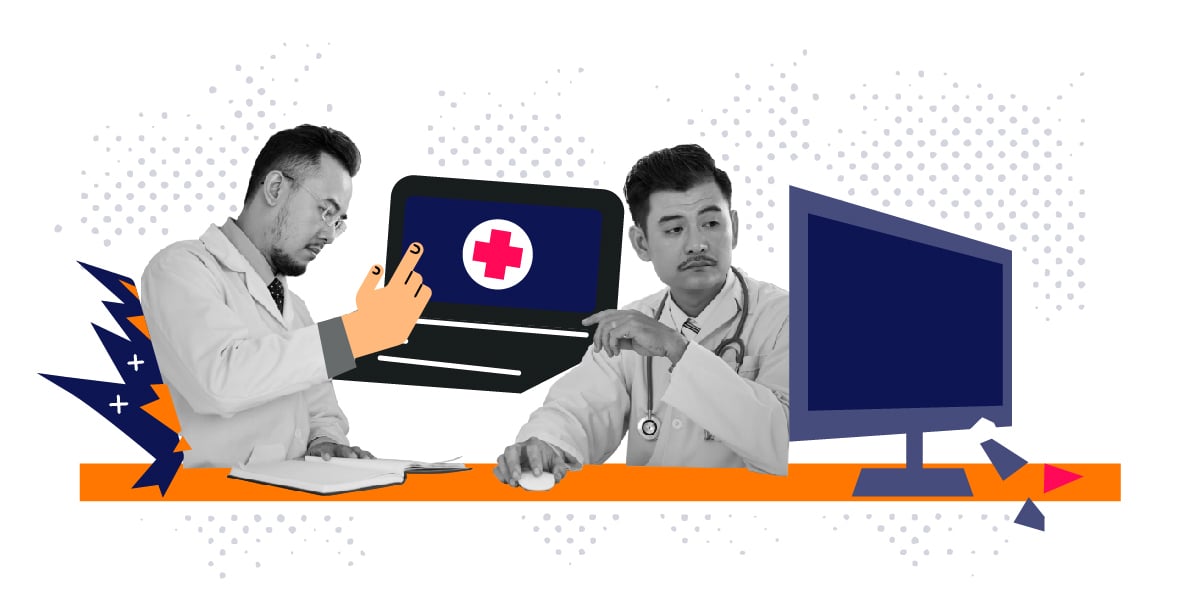
- Autonomous Vehicles: Consider autonomous vehicles as a prime example of successful hybrid AI integration. Image recognition, a form of Narrow AI, identifies objects and obstacles, while General AI’s contextual understanding enables the vehicle to interpret complex road scenarios. This fusion ensures the recognition of objects and comprehension of their context—an imperative for safe navigation.
- Healthcare Diagnostics: In the realm of healthcare, successful hybrid AI models enhance diagnostic accuracy. Image recognition (Narrow AI) analyzes medical images with precision, while adaptability and learning capacities (General AI) ensure a broader understanding of patient health. The result is a marriage of specialization and adaptability, marking a significant advancement in medical diagnostics.
- Virtual Assistants: Virtual assistants exemplify the successful integration of Weak AI’s mimicry and Strong AI’s potential for comprehension. These assistants mimic human-like interactions (Weak AI) while incorporating elements of comprehension and adaptability (Strong AI), offering users a seamless and dynamic experience.
In understanding these fundamental types of AI, enterprises gain a foundational grasp of the diverse landscapes within the field. The distinctions between Narrow AI, General AI, and Weak AI and Strong AI serve as compass points for decision-makers navigating the complexities of AI adoption. As we continue our exploration into the functionalities and capabilities of AI, these fundamental types lay the groundwork for enterprises to align their strategies with the vast potential of intelligent technologies.
Hybrid AI models exemplify the synergy achievable by combining different AI types. Enterprises exploring AI solutions can draw inspiration from these success stories, recognizing the potential for customized, practical applications.
AI Classified Based on Functionality
In the expansive world of artificial intelligence (AI), the classification based on functionality serves as a roadmap, guiding your enterprise through the diverse applications and operational modes of intelligent systems.
Now, we’ll gain essential insights into the functional aspects of AI, understand how systems operate in real-time decision-making, and explore cutting-edge models that aim to instill AI with human-like cognitive understanding.
Reactive Machines in AI
Another lens through which to understand AI is functionality. Reactive Machines, a type of AI, operate based on predefined rules and respond to specific inputs with predetermined actions. While unable to learn from new scenarios, Reactive Machines excel in real-time decision-making.
At the core of AI functionality lies Reactive Machines, systems that operate based on predefined rules and responses. These systems excel in real-time decision-making, quickly analyzing inputs and executing predetermined actions. Unlike learning-oriented models, Reactive Machines don’t evolve with experience but rely on fixed algorithms to navigate dynamic scenarios.
In applications like chess-playing programs, Reactive Machines showcase their prowess. By evaluating possible moves and selecting the optimal one based on preprogrammed rules, these systems exhibit a remarkable capacity for strategic decision-making in dynamic environments.
Impact on Real-time Decision-making
The immediate impact of Reactive Machines is most evident in scenarios where quick decision-making is paramount. Chess-playing programs, for instance, employ Reactive Machines to evaluate potential moves and select the optimal one based on preprogrammed rules. This instantaneous decision-making capability is a cornerstone in applications demanding swift responses.
Examples of Reactive Machines
Consider automated trading systems in finance, where Reactive Machines implement predefined algorithms to execute trades based on market conditions. The ability to make split-second decisions aligns with the precision required in dynamic financial markets, showcasing the practical application of Reactive Machines in real-world scenarios.
Automated Customer Support Chatbot
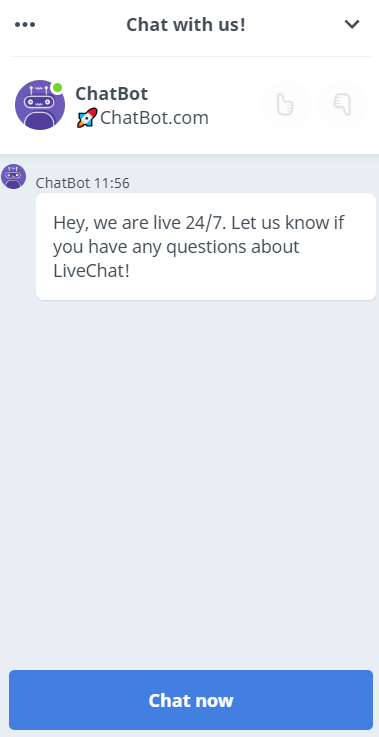
- Description: Many businesses use automated customer support chatbots that operate as reactive machines. These chatbots are designed to respond to user queries based on predefined rules and patterns. They analyze the input from customers and provide responses or take actions according to a set of programmed instructions.
- Implementation:
- Rule-Based Responses: The chatbot follows predetermined rules to address common customer queries.
- FAQ Matching: It matches user input with a database of frequently asked questions and provides relevant answers.
- Limited Context Awareness: The chatbot lacks a comprehensive understanding of customer emotions or long-term context but is effective in handling routine inquiries.
Personalized Marketing Recommender System
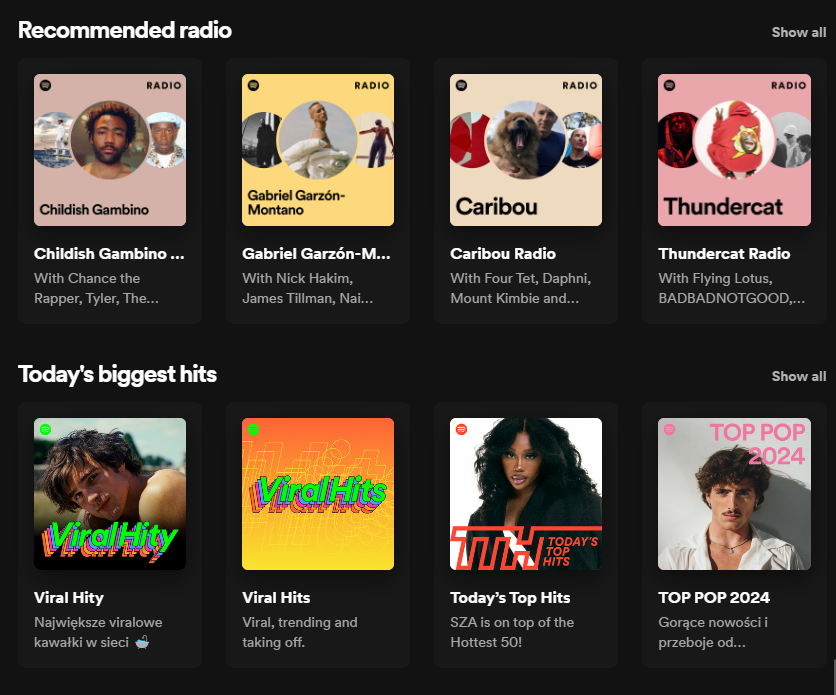
- Description: A Theory of Mind model can be applied in a business context to enhance personalized marketing recommendations. The system aims to understand the customer’s preferences, emotions, and purchasing intent, adapting its recommendations based on a more nuanced understanding of the customer’s mental states.
- Implementation:
- User Behavior Analysis: The system analyzes past user behavior, including purchase history, browsing patterns, and interaction with marketing content.
- Sentiment Analysis: Incorporating sentiment analysis, the model interprets customer reviews, feedback, and social media interactions to gauge current emotional states.
- Adaptive Recommendations: The system dynamically adjusts product recommendations, marketing messages, and promotions based on its evolving understanding of the customer’s preferences and emotional context.
These examples showcase how Reactive Machines and Theory of Mind models can be integrated into business processes to enhance customer interaction, support, and personalization, each serving a specific purpose in meeting business objectives.
Theory of Mind Models
Advancing the classification based on functionality, Theory of Mind models bring a new dimension to AI functionality. Inspired by human cognition, these models aim to imbue AI with an understanding of human emotions, intentions, and beliefs.
However, implementing Theory of Mind models introduces ethical considerations, raising questions about privacy and the potential manipulation of user emotions. Imagine an AI-powered virtual assistant capable of gauging user emotions and adjusting its responses accordingly. While this promises a more personalized and empathetic user experience, it also demands a careful balance to avoid ethical pitfalls.
Ethics in Implementation
Implementing Theory of Mind models introduces ethical considerations concerning privacy and the potential manipulation of user emotions. As AI systems delve into understanding and responding to human emotions, the responsible deployment of such capabilities becomes paramount to ensure user trust and data integrity.
Example of Theory of Mind Models
E-commerce Customer Engagement Platform
- Description: An e-commerce platform may implement a combination of Reactive Machines and Theory of Mind models. The platform’s chatbot, acting as a reactive machine, handles basic customer queries efficiently. Simultaneously, the system employs a Theory of Mind model to personalize product recommendations and promotional messages based on the customer’s past behavior, preferences, and real-time sentiment.
- Implementation:
- Chatbot Integration: The chatbot quickly addresses common inquiries such as order status, shipping information, and return policies using reactive rules.
- Personalized Recommendations: The system, equipped with a Theory of Mind model, analyzes customer behavior, understands preferences, and tailors product recommendations through machine learning algorithms.
- Feedback Loop: The platform continuously learns from user interactions, refining its understanding of individual preferences and improving both reactive and proactive customer engagement.
AI Grouped According to Capabilities
AI shapes the digital landscape, but instead of being a monolithic entity, it represents a spectrum of capabilities that cater to distinct needs and operational paradigms.
Navigating the diverse landscape of artificial intelligence involves understanding how these intelligent systems operate based on their capabilities. Two prominent categories exist. These include Assisted Intelligence and Autonomous Intelligence.
Assisted Intelligence is designed to augment human capabilities, while Autonomous Intelligence represents AI systems with remarkable self-sufficiency.
Assisted Intelligence to Augment Human Capabilities
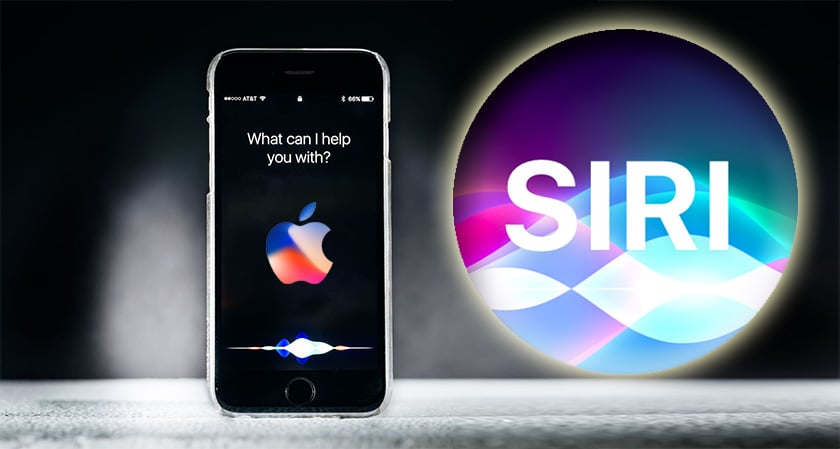
As AI evolves, Assisted Intelligence emerges as a category designed to augment human capabilities rather than replace them. It’s a neat example of the symbiotic relationship between humans and machines. These AI systems adapt to user needs, providing support and enhancing efficiency. Chatbots in customer service and productivity tools that automate repetitive tasks are examples of Assisted Intelligence in action.
Adaptation to User Needs
At the core of Assisted Intelligence is its remarkable ability to adapt to user requirements. Whether in customer service interactions or productivity tools automating routine tasks, these AI systems function harmoniously with human users, understanding their needs and providing tailored assistance.
Real-world Examples Displaying User Needs
Consider virtual collaboration tools equipped with Assisted Intelligence. These tools anticipate user needs, automate routine tasks, and streamline interactions, allowing teams to concentrate on creative problem-solving. The adaptability of Assisted Intelligence positions it as a valuable asset in diverse industries, enhancing operational efficiency and elevating user experiences.
For enterprises seeking to streamline operations and improve user experiences, Assisted Intelligence offers a tailored solution. The adaptability of these systems to specific user requirements positions them as valuable assets in diverse industries.
Balancing Automation and Collaboration
The essence of Assisted Intelligence lies in striking the delicate balance between automation and collaboration. By automating repetitive tasks and providing real-time support, these systems empower users to focus on tasks that demand human creativity and critical thinking. In doing so, Assisted Intelligence becomes a catalyst for productivity and innovation.
Applications of Assisted AI in Customer Service
In customer service, chatbots powered by Assisted Intelligence engage users in natural language conversations, swiftly addressing queries and providing relevant information. This dynamic interaction enhances customer satisfaction and frees human agents to tackle more complex and nuanced customer issues.
Autonomous Intelligence
On the other end of the spectrum, Autonomous Intelligence represents AI systems operating with high self-sufficiency. Autonomous Intelligence is reshaping industries from self-driving cars navigating complex road conditions to drones executing precision tasks.
These systems, characterized by autonomy in decision-making and execution, have the potential to reshape industries by reducing reliance on continuous human intervention.
Autonomous Intelligence embodies systems capable of making decisions and executing tasks without constant human oversight. The self-sufficiency of these systems positions them at the forefront of technological innovation, offering the promise of increased efficiency and operational autonomy.
Essential Examples of Autonomous AI in the Industry
Consider the revolutionary impact of Autonomous Intelligence in industries such as transportation. Autonomous vehicles, equipped with advanced AI algorithms, navigate complex road conditions, make split-second decisions, and redefine the future of transportation. Beyond roads, drones with Autonomous Intelligence execute precision tasks in various sectors, from agriculture to logistics.
Challenges in Widespread Adoption of Autonomous Intelligence
While the transformative potential of Autonomous Intelligence is undeniable, widespread adoption faces challenges. Regulatory frameworks must adapt to accommodate these self-sufficient systems, addressing concerns related to safety, ethical considerations, and the need for fail-safe mechanisms.
Balancing Autonomy and Safety
The successful integration of Autonomous Intelligence requires a delicate balance between autonomy and safety. Innovations in sensor technologies, robust algorithms, and real-time decision-making capabilities are crucial in ensuring the safe deployment of autonomous systems.
This is a good place to review Tesla’s self-driving car journey.
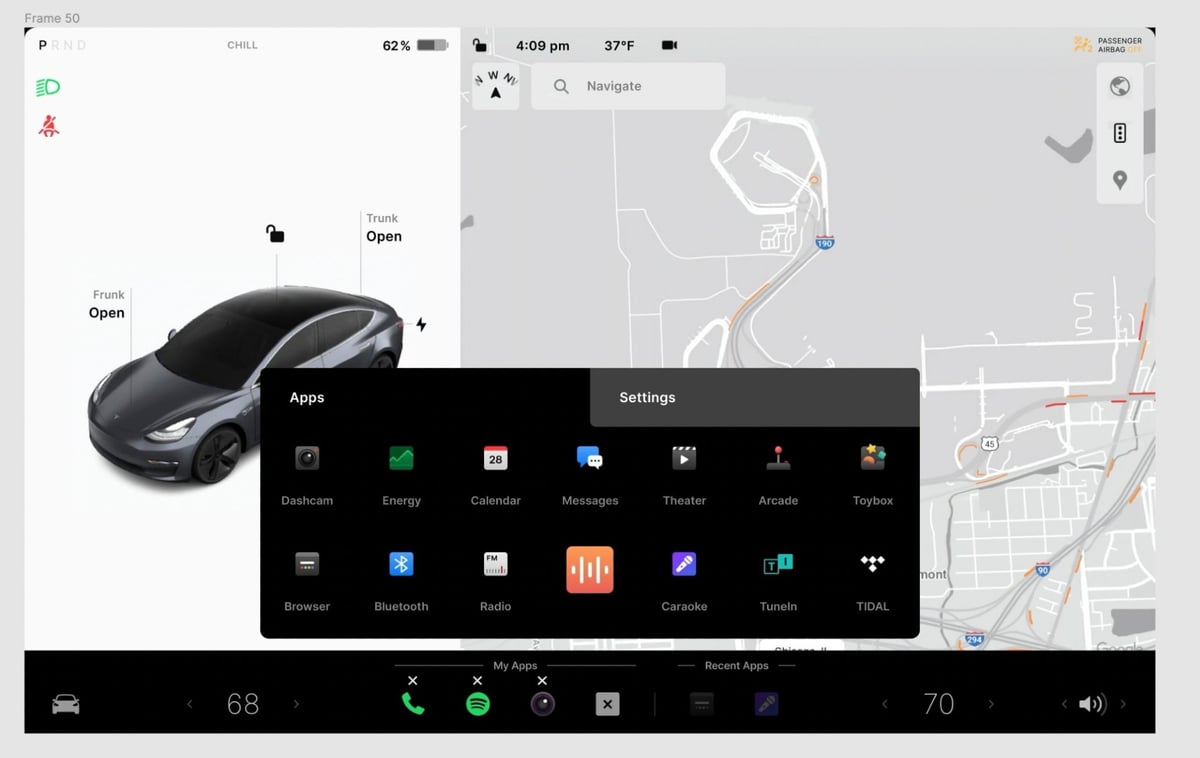
The company has faced challenges and controversies regarding its autonomous AI technology, particularly its Full Self-Driving (FSD) capabilities. Critics have raised concerns about the deployment of beta versions to a wide user base, highlighting instances of the system’s limitations and occasional erratic behavior.
Tesla’s approach of relying heavily on real-world data gathered from its fleet of vehicles for training the AI has sparked debates about safety and the need for more traditional simulation-based testing. Additionally, regulatory bodies and industry experts have emphasized the importance of comprehensive safety assessments for autonomous systems.
While Tesla continues to make strides in autonomous technology, its struggles include addressing safety concerns, regulatory compliance, and the need for robust testing methodologies to ensure the reliability of its AI systems in diverse and complex driving scenarios. For the latest updates, it is advisable to refer to more recent sources.
Applications Beyond the Transport Industry
Autonomous Intelligence extends its reach beyond transportation, finding applications in scenarios where continuous, independent decision-making is advantageous. From automated warehouse systems optimizing logistics to robotic systems performing intricate surgeries, the impact of Autonomous Intelligence is pervasive and transformative.
While the potential benefits are immense, widespread adoption faces challenges, including regulatory hurdles and safety concerns. Navigating these challenges is crucial for enterprises eyeing the transformative potential of Autonomous Intelligence.
Choosing the Right AI Fit for Your Business
As enterprises navigate the dynamic landscape of AI capabilities, the choice between Assisted Intelligence and Autonomous Intelligence becomes pivotal. The decision hinges on three things:
- The nature of tasks.
- The level of autonomy required.
- The desired connection between human and machine capabilities.
Tailoring Solutions with Assisted Intelligence
For tasks requiring collaboration, adaptability, and human-machine partnership, Assisted Intelligence appears as the ideal choice. Its ability to understand user needs, provide real-time support, and enhance efficiency positions it as a valuable ally in industries where human creativity and decision-making are indispensable.
Transformative Potential of Autonomous Intelligence
In sectors demanding operational autonomy and swift decision-making, the transformative potential of Autonomous Intelligence comes out. From reshaping transportation with self-driving vehicles to revolutionizing logistics with autonomous drones, the impact is profound, offering unparalleled efficiency and innovation.
Blending Assisted Intelligence and Autonomous Intelligence
The optimal AI solution for your company lies in leveraging the connection between Assisted Intelligence and Autonomous Intelligence. This hybrid approach combines the adaptability and collaboration of Assisted Intelligence with the self-sufficiency and efficiency of Autonomous Intelligence, creating a harmonious blend that addresses diverse enterprise needs.
Hybrid Solutions for Complex Challenges
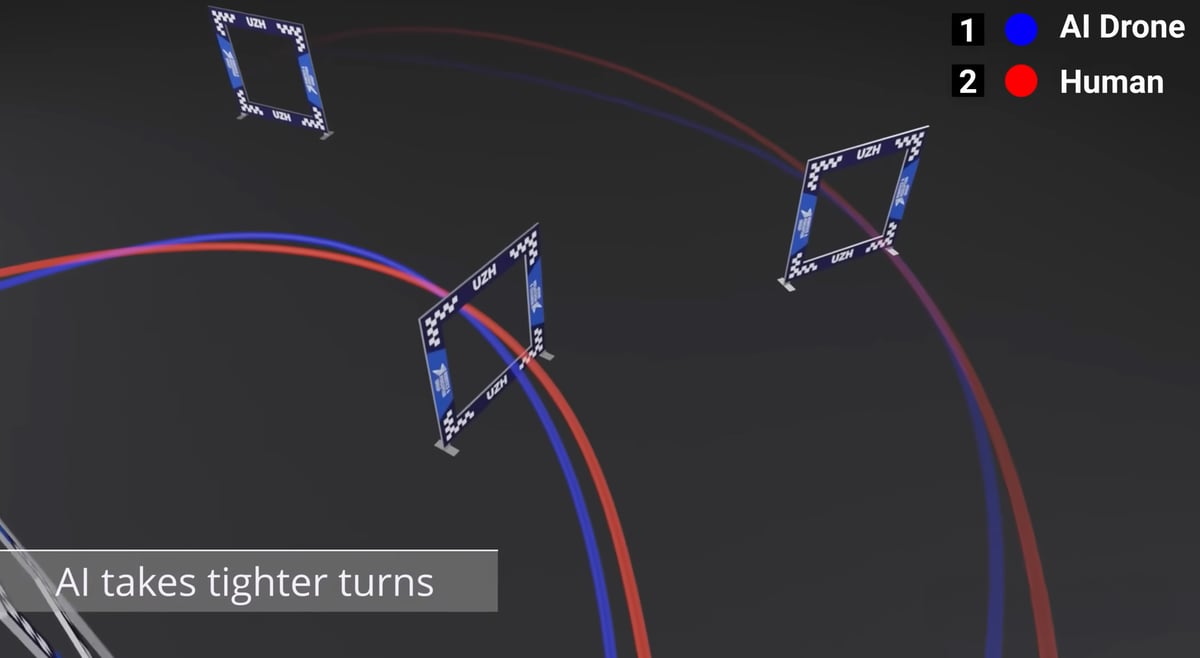
Consider scenarios where autonomous drones equipped with AI collaborate with human operators for tasks requiring a balance between autonomy and human oversight. This collaborative synergy extends to various industries, including search and rescue missions, environmental monitoring, and infrastructure inspections.
Navigating the Future of AI Capabilities
As AI capabilities evolve, enterprises must strategically navigate the choices presented by Assisted Intelligence and Autonomous Intelligence. The future holds the promise of increasingly sophisticated systems seamlessly integrating with human operations and reshaping industries across the spectrum.
Navigating the future of AI capabilities requires a strategic and ethical approach that balances innovation with responsible deployment. As AI technologies continue to evolve, businesses and policymakers must collaborate to establish clear ethical guidelines, regulations, and standards. Investments in research and development, alongside fostering a diverse talent pool, will be essential to unlock the full potential of AI.
Interdisciplinary collaboration, involving experts from various fields, will be crucial in addressing challenges related to bias, transparency, and accountability. Emphasizing continuous learning and adaptability will be key as AI capabilities advance, ensuring that organizations can harness the technology effectively, ethically, and in alignment with societal values.
Building a robust framework for AI governance, promoting transparency, and fostering public understanding will be integral components of successfully navigating the future landscape of AI capabilities.
Understanding the classification of AI based on capabilities empowers decision-makers to align AI solutions with specific enterprise needs. Whether augmenting human capabilities through collaboration or ushering in a new era of autonomous operations, the diverse landscape of AI capabilities offers unprecedented opportunities for innovation and efficiency.
AI Types Based on Learning Mechanisms
Diving into the intricate artificial intelligence (AI) world, this section unveils the diverse learning mechanisms underpinning intelligent systems. From the linchpin role of Supervised Learning in pattern recognition to the autonomous exploration of patterns in Unsupervised Learning and the dynamic adaptation of Reinforcement Learning in complex environments, we explore how these mechanisms drive AI’s learning and decision-making capabilities, shaping its role in diverse real-world scenarios.
Supervised Learning
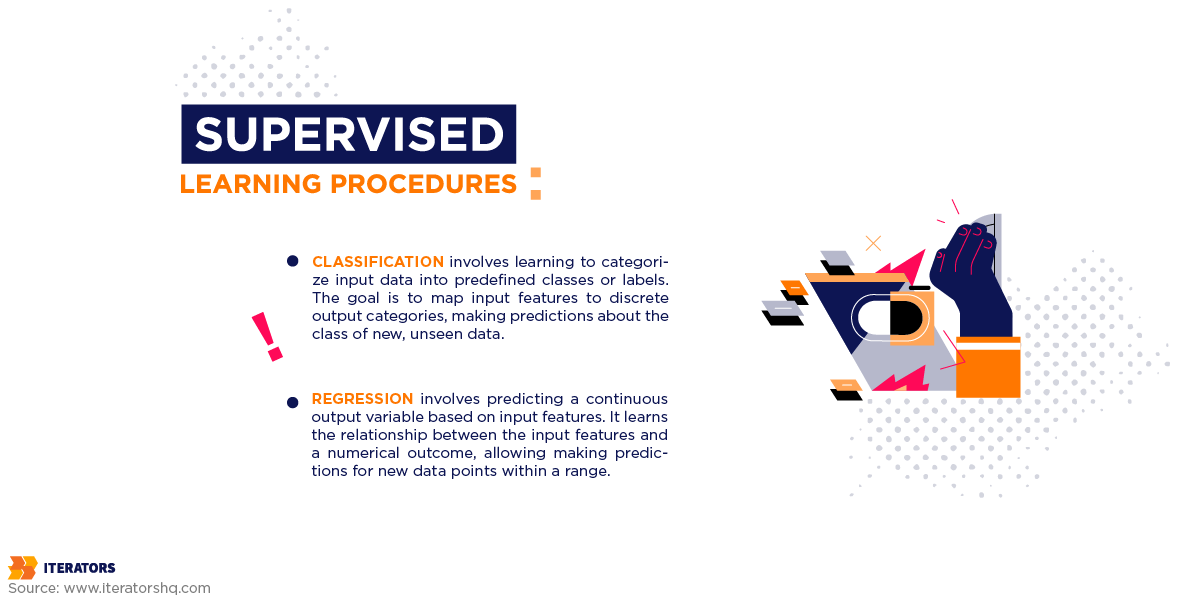
In terms of learning mechanisms, Supervised Learning serves as a linchpin. This approach involves training an AI model on labeled data, allowing it to learn patterns and make highly accurate predictions. Applications of supervised learning range from image recognition in healthcare to fraud detection in finance.
Role and Efficacy of Supervised Learning
Supervised Learning’s efficacy is evident as a foundational building block for various AI applications. It’s similar to having a mentor guide their protege using many examples.
The model learns to associate inputs with correct outputs, making accurate predictions when encountering new and previously unseen data.
Supervised learning is especially effective in applications where precision and reliability are a priority. Enterprises can leverage this approach to create solutions tailored to their specific data sets and requirements.
Successful Implementations of Supervised Learning
Numerous breakthroughs underscore the efficacy of Supervised Learning, especially in image recognition. Models trained on vast datasets with labeled images accurately identify objects, faces, and intricate patterns.
The success of these applications is a testament to the power of Supervised Learning in discerning complex relationships within data.
Enterprises leveraging Supervised Learning witness tailored solutions aligned with specific data sets and requirements. For instance, AI models trained through Supervised Learning enhance diagnostic accuracy in medical diagnostics by identifying subtle patterns indicative of various medical conditions. This precision has transformative implications for healthcare, offering efficient and accurate diagnostic capabilities.
Adaptability of Supervised Learning Across Industries
The adaptability of Supervised Learning transcends specific domains. From natural language processing for chatbots to predicting financial market trends, its applications are diverse and impactful.
By providing clear guidelines through labeled data, Supervised Learning empowers AI systems to navigate complex decision-making scenarios with a human-like understanding.
Challenges and Outlook of Supervised Learning Development
While Supervised Learning excels in scenarios with labeled datasets, challenges arise when dealing with unstructured or unlabeled data. Addressing these challenges involves refining algorithms and exploring hybrid approaches that leverage the strengths of multiple learning mechanisms. The future holds the promise of enhanced adaptability as Supervised Learning continues to evolve in tandem with the ever-changing landscape of AI.
Unsupervised Learning
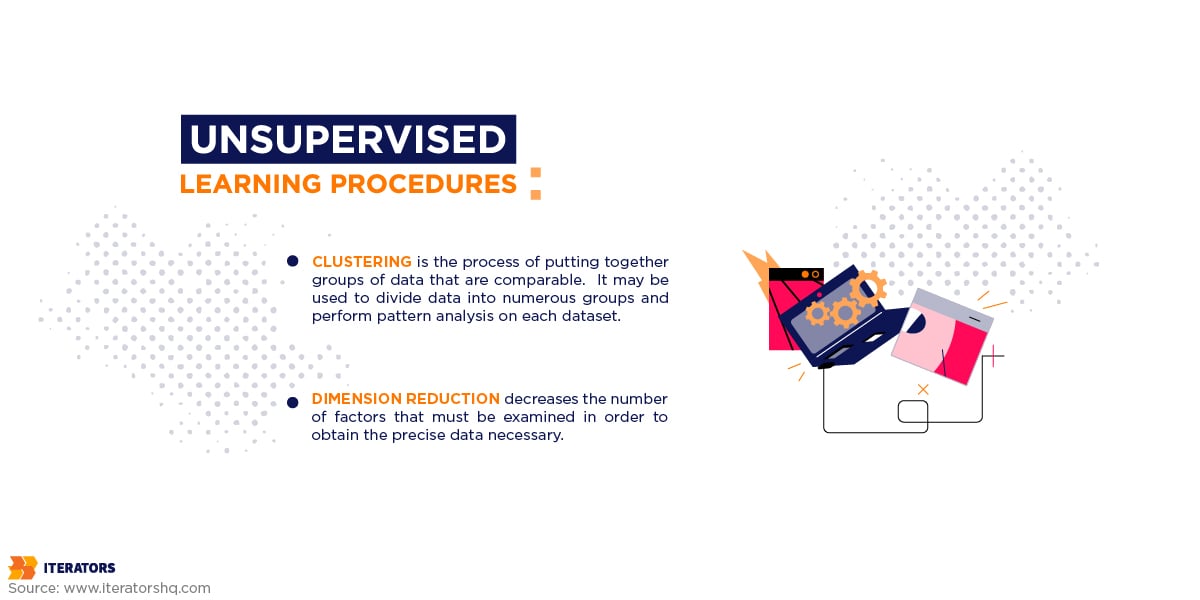
Unsupervised Learning is a pioneering approach for navigating massive unlabeled data. It enables AI systems to identify patterns and relationships autonomously. This approach is beneficial when dealing with large datasets where manually labeling data is impractical.
Working without predefined output labels, unsupervised learning allows your AI systems to autonomously explore and identify hidden patterns and relationships.
Navigating the potential challenges, such as the need for robust algorithms and careful data preprocessing, allows enterprises to unlock the full potential of Unsupervised Learning in uncovering hidden insights within their data.
Key Notes of Unsupervised Learning Solutions
The distinguishing characteristic of Unsupervised Learning lies in its ability to uncover inherent structures within data without explicit guidance.
In scenarios where labeling vast datasets is impractical, Unsupervised Learning becomes instrumental in extracting meaningful insights and identifying patterns that might elude human observation.
Potential Issues with Unsupervised Learning
While the autonomy of Unsupervised Learning is its strength, navigating potential challenges is crucial. Without predefined labels, the system must discern meaningful patterns and relationships, which can pose difficulties in noisy or ambiguous data scenarios.
Robust algorithms and careful data preprocessing are essential to ensure optimal outcomes.
Effectiveness of Unsupervised Learning
Unsupervised Learning finds its application in diverse real-world scenarios. Consider marketing strategies where customer segmentation is pivotal. Unsupervised Learning excels in categorizing customers based on behavior, revealing patterns that inform targeted marketing approaches.
This versatility extends to fields such as anomaly detection, where the system identifies deviations from expected patterns in data.
Data-Driven Discoveries with Unsupervised Learning
At its core, Unsupervised Learning empowers data-driven discoveries. In scientific research, for example, it plays a crucial role in identifying patterns in genomic data or uncovering hidden relationships in complex biological systems. By allowing the system to navigate the unlabeled terrain autonomously, Unsupervised Learning becomes a catalyst for groundbreaking insights.
Challenges and Future Trajectories
While Unsupervised Learning presents a formidable tool for uncovering hidden insights, challenges persist in high-dimensional or sparse data scenarios. The evolution of this learning mechanism involves addressing these challenges through hybrid models that combine the strengths of both Supervised and Unsupervised Learning. The future trajectory of Unsupervised Learning holds promise, mainly as AI systems aim to extract actionable knowledge from vast and complex datasets autonomously.
Reinforcement Learning
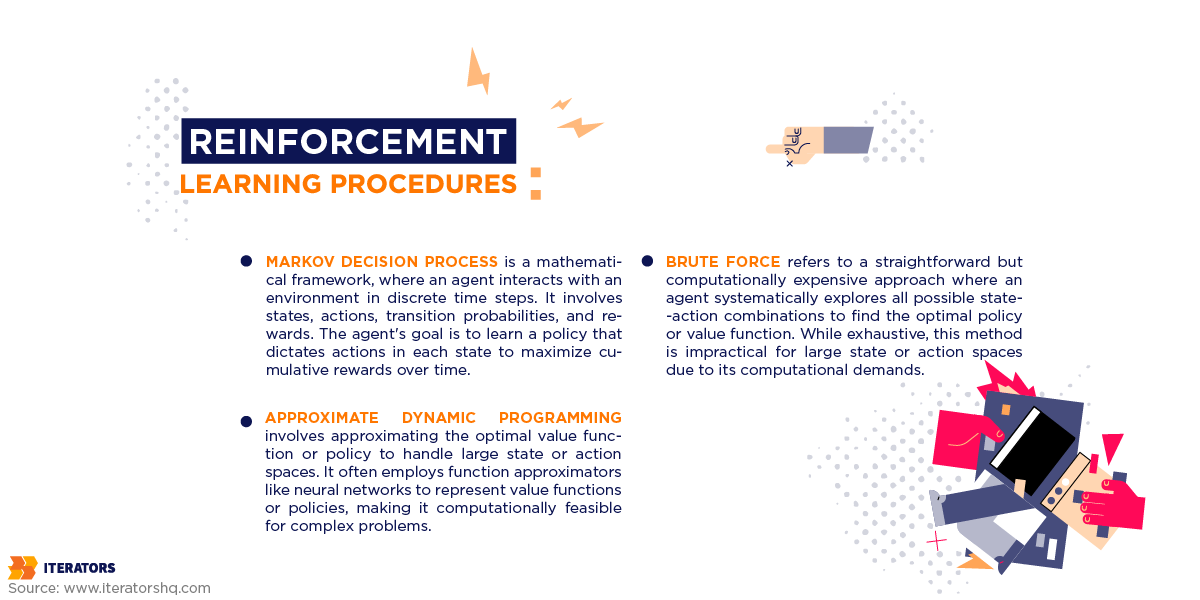
Reinforcement Learning is especially suited for scenarios demanding dynamic adaptation in complex environments. It mimics how humans learn through trial and error, with an AI agent receiving feedback as rewards or penalties based on actions, allowing it to optimize decision-making progressively.
Practical Benefits of Reinforcement Learning
Reinforcement Learning shines in practical scenarios where continuous interaction with the environment is essential. The iterative process of trial and error enables the AI system to learn optimal behaviors, adapt to changing conditions, and refine its strategies over time. This adaptability positions Reinforcement Learning as a preferred approach in fields such as robotics, gaming, and autonomous systems.
Success Stories in Complex Environments
The success of Reinforcement Learning is evident in its application to train robots for intricate tasks. In manufacturing, for example, robots can learn to precisely manipulate objects through repeated attempts, refining their movements based on feedback. The adaptability showcased in these environments underscores the potential of Reinforcement Learning in addressing complex challenges.
Reinforcement learning has found success in various business applications, showcasing its ability to optimize decision-making processes and adapt to dynamic environments. Here are five successful use cases of reinforcement learning in business:
- Autonomous Systems and Robotics:
Example: Warehouse Management
Description: Reinforcement learning is applied to optimize the movement of autonomous robots within warehouses. Robots learn to navigate efficiently, avoid obstacles, and optimize pick-and-place operations. This enhances overall warehouse productivity and reduces operational costs. - Finance and Algorithmic Trading:
Example: Stock Trading
Description: Reinforcement learning algorithms are employed in algorithmic trading systems to adapt trading strategies based on market conditions. These systems learn from historical data and real-time market changes to make optimal trading decisions, maximizing returns while minimizing risks. - Recommendation Systems:
Example: Content Recommendation
Description: Reinforcement learning is used in recommendation systems to personalize content suggestions. By learning user preferences and feedback over time, these systems can dynamically adjust recommendations, improving user engagement and satisfaction in platforms such as streaming services or e-commerce websites. - Marketing and Dynamic Pricing:
Example: Dynamic Pricing in E-commerce
Description: Reinforcement learning is applied to determine optimal pricing strategies in real-time. The algorithm learns from user behavior, market demand, and competitor pricing to adjust product prices dynamically, maximizing revenue and maintaining competitiveness. - Supply Chain Optimization:
Example: Inventory Management
Description: Reinforcement learning is utilized in supply chain management to optimize inventory levels. Algorithms learn from historical demand patterns, supplier performance, and other variables to make real-time decisions, minimizing stockouts and excess inventory, ultimately reducing costs and improving efficiency.
These use cases demonstrate the versatility of reinforcement learning across various industries, showcasing its ability to adapt and optimize decision-making processes in dynamic and complex business environments.
Balancing Exploration and Exploitation
One key aspect of Reinforcement Learning is the delicate balance between exploration and exploitation. The AI agent explores various actions to understand their outcomes, utilizing this information to make informed decisions. This constant interplay ensures that the system learns from successes and explores novel strategies to discover potentially better solutions.
Real-world Applications of Reinforcement Learning
Practical applications of Reinforcement Learning span diverse industries. In finance, AI algorithms utilizing Reinforcement Learning can optimize trading strategies by learning from market dynamics and adjusting approaches based on historical performance. This adaptability proves invaluable in navigating the complex and ever-changing landscape of financial markets.
Challenges and Continuous Learning
While Reinforcement Learning excels in scenarios requiring continuous adaptation, challenges persist in scaling the approach to handle high-dimensional or continuous state spaces. Additionally, issues related to sample efficiency and the need for extensive training data are areas of ongoing research. The continuous learning aspect of Reinforcement Learning positions it as a field of exploration, with continued efforts to refine its capabilities.
Potential Trajectories and Industry Impact
The future trajectories of Reinforcement Learning hold promise, especially as industries seek AI systems capable of autonomous decision-making in dynamic environments. From optimizing resource allocation in logistics to enhancing control systems in autonomous vehicles, the impact of Reinforcement Learning extends across sectors, promising innovative solutions to complex challenges.
In specific scenarios where continuous interaction with the environment is essential, Reinforcement Learning is preferable. This mechanism involves an AI agent learning through trial and error receiving feedback through rewards or penalties.
Consider the application of Reinforcement Learning in training robots to perform complex tasks. By allowing the system to learn optimal behaviors through experimentation, enterprises can deploy solutions that adapt dynamically to changing conditions.
Specific Types of AI Applications
As you venture into building your artificial intelligence applications, understanding how AI seamlessly integrates into various landscapes is necessary. From the transformative influence of Natural Language Processing (NLP) to the distinct capabilities of Computer Vision and the advancements in Speech Recognition, we’ll now unravel the real-world impact of these specific AI applications.
Natural Language Processing (NLP)
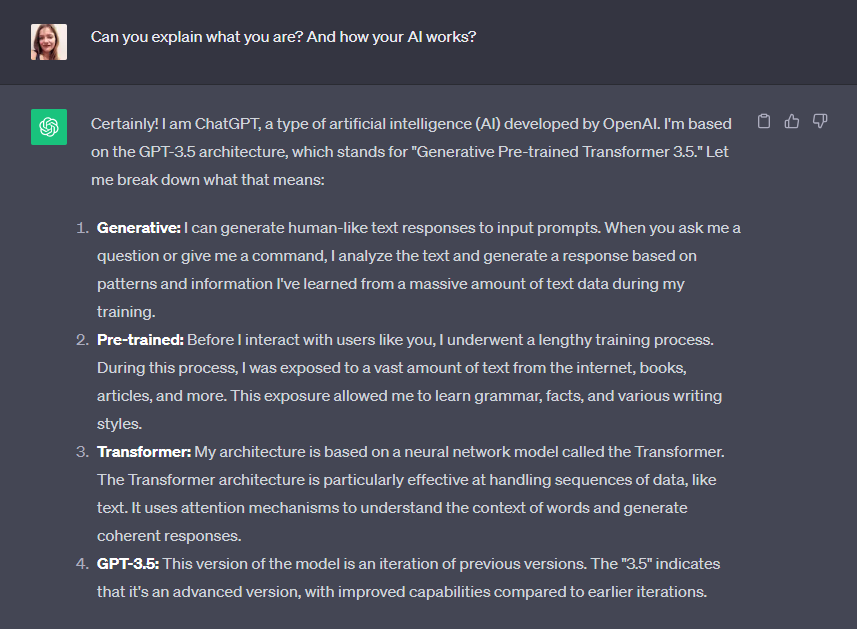
Among the diverse applications, Natural Language Processing (NLP) seamlessly integrates into the AI landscape, enabling machines to understand and respond to human language. Recent advancements, such as OpenAI’s GPT-3, have propelled NLP to new heights, showcasing its potential to generate human-like text and powering chatbots that engage in natural conversations.
Amazon and Google are two companies using NLP in their operations:
- Google:
Application: Google Search and Google Assistant
Description: Google extensively employs NLP in its search engine algorithms to understand and interpret user queries more accurately. Google’s search algorithms use NLP techniques to comprehend the context, intent, and semantics of search queries, delivering more relevant search results. Additionally, Google Assistant, the virtual assistant developed by Google, relies on NLP for natural language understanding, enabling users to interact with their devices using voice commands in a conversational manner. - Amazon:
Application: Alexa and Amazon Comprehend
Description: Amazon’s voice-activated virtual assistant, Alexa, leverages NLP to understand and respond to user voice commands. Alexa uses NLP algorithms to process and interpret spoken language, enabling tasks such as setting reminders, answering questions, and controlling smart home devices. Moreover, Amazon Comprehend, a natural language processing service provided by Amazon Web Services (AWS), is designed to extract insights and sentiment from large volumes of text data, supporting businesses in analyzing customer reviews, feedback, and other textual information.
There’s a significant increase in the accuracy of language models, with NLP becoming a cornerstone in applications ranging from virtual assistants to content generation.
Computer Vision (CV)
Considered a distinct and transformative AI application, Computer Vision enables machines to interpret and make decisions based on visual data. Industries such as healthcare, where diagnostic imaging benefits from AI-driven analysis, showcase the transformative potential of Computer Vision.
Like Tesla, Amazon also uses Computer Vision to good effect as follows:
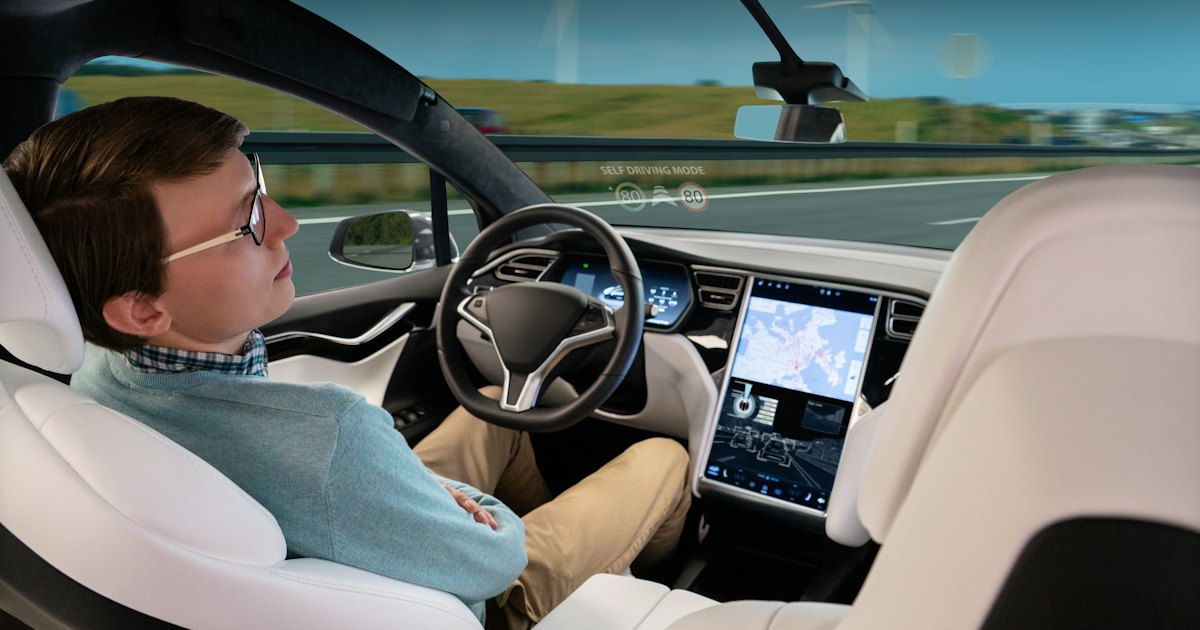
- Tesla:
Application: Autopilot System
Description: Tesla, the electric car manufacturer, employs Computer Vision as a core technology in its Autopilot system. Cameras and sensors integrated into Tesla vehicles capture real-time visual data from the environment. Computer Vision algorithms then process this data to identify objects, lane markings, and other relevant features on the road. The Autopilot system utilizes Computer Vision to enable features such as adaptive cruise control, automatic lane-keeping, and collision avoidance. - Amazon:
Application: Amazon Go Stores
Description: Amazon utilizes Computer Vision in its cashier-less Amazon Go stores. Computer Vision algorithms, combined with sensors and cameras installed in the store, track customers as they pick up items from shelves. The system automatically adds the selected items to the customer’s virtual cart, and upon exiting the store, the customer’s account is charged for the items they took. This application of Computer Vision eliminates the need for traditional checkout processes, providing a seamless and convenient shopping experience.
Real-world examples underline the impact of Computer Vision, with facial recognition technology revolutionizing security systems and object recognition enhancing manufacturing processes.
Speech Recognition
AI in Speech Recognition has made significant strides towards achieving human-level accuracy, contributing to the evolution of human-machine interaction. Speech Recognition has become an integral part of daily life, from voice-activated assistants to transcription services.
Microsoft and Nuance Communications use speech recognition to offer gravity-defying product experiences:
- Microsoft:
Application: Microsoft Azure Speech Services
Description: Microsoft offers Speech Recognition capabilities through its Azure Speech Services. These services enable organizations to integrate speech-to-text and text-to-speech capabilities into their applications. Companies can use Microsoft’s Speech Recognition technology for various applications, including voice commands in applications, transcription services, and voice-enabled virtual assistants. - Nuance Communications:
Application: Dragon NaturallySpeaking
Description: Nuance Communications specializes in speech and imaging applications, and its Dragon NaturallySpeaking software is a prominent example of Speech Recognition technology. Dragon NaturallySpeaking allows users to dictate text and control various applications using voice commands. It is widely used in professional settings, such as healthcare, legal, and business environments, where accurate and efficient Speech Recognition is crucial for documentation and workflow optimization.
Advancements in neural networks and deep learning algorithms have propelled Speech Recognition technologies, making them more accessible and reliable for diverse applications.
Classifying AI in Terms of Complexity
Let us now consider the intricacies of classifying AI by complexity. From the streamlined functionality of Simple AI systems in everyday tasks to the intricate handling of Complex AI models in specialized domains, we navigate the correlation between complexity and real-world applications.
The correlation between AI complexity and real-world applications is critical for enterprises. At the same time, simple AI systems excel in specific tasks, and complex models open doors to addressing multifaceted challenges.
Research findings indicate that the appropriateness of simplicity or complexity depends on the specific use case. Striking a balance ensures optimal usability, making AI solutions more accessible and effective.
Simple AI Systems
In terms of complexity, Simple AI Systems find practical utility in everyday tasks. These systems, often rule-based and focused on specific functions, excel in scenarios where simplicity and efficiency are paramount.
Here are two examples of applications using Simple AI systems:
- Spam Filters in Email:
Application: Email Filtering
Description: Spam filters are a common example of Simple AI systems that use rule-based algorithms and machine learning techniques to identify and filter out unwanted emails (spam). These systems analyze the content, sender information, and various other features of emails to determine whether they are likely to be spam or legitimate. Over time, they learn from user feedback to continuously improve their accuracy in distinguishing between spam and non-spam emails. - Autocorrect in Text Processing:
Application: Keyboard Auto Correction
Description: Autocorrect features in text processors and mobile devices are Simple AI systems that correct spelling errors and predict the intended words based on contextual information. These systems use algorithms to analyze the sequence of typed characters, compare them to a dictionary, and suggest corrections or completions. Autocorrect mechanisms help improve the accuracy and efficiency of text input by users.
These examples demonstrate how Simple AI systems can be applied to streamline specific tasks and enhance user experiences in everyday applications. They are tailored to perform well-defined functions, making them efficient for specific use cases without requiring the broader capabilities associated with general artificial intelligence.
Complex AI Models
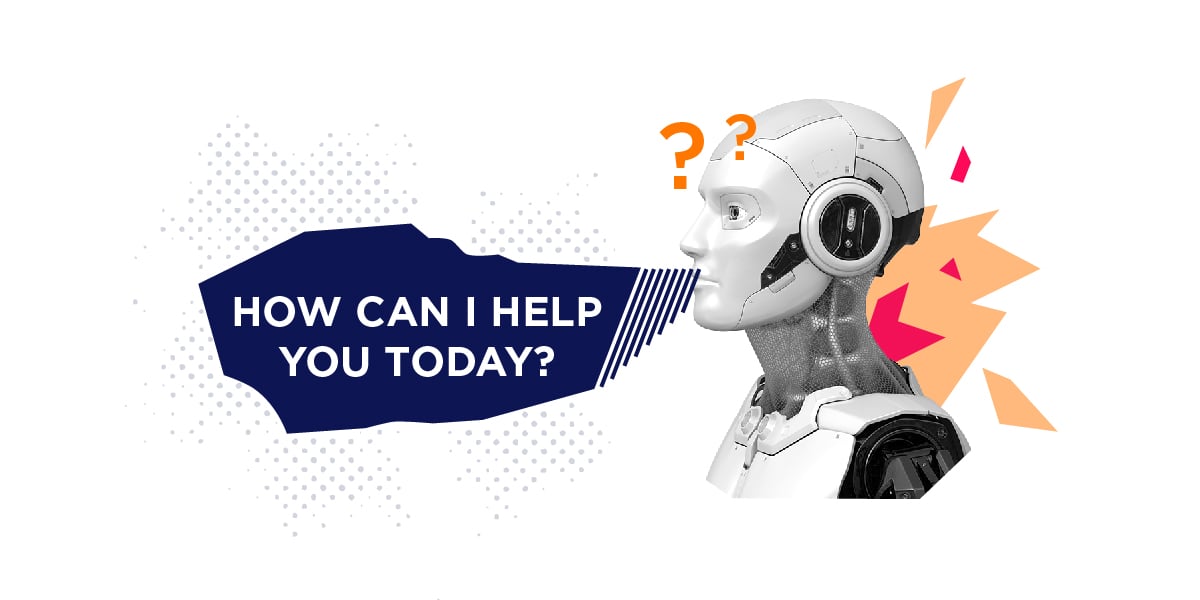
Contrastingly, Complex AI Models tackle intricate tasks and data. Their ability to handle complexity positions them as powerful tools for solving complex problems, such as weather prediction or financial modeling.
Navigating the scalability considerations inherent in complex models is vital for real-world deployment. Balancing sophistication with practicality ensures that these models contribute meaningfully to problem-solving.
Here are two examples of applications using Complex AI models:
- Image Recognition in Healthcare:
Application: Medical Image Analysis
Description: Complex AI models, such as convolutional neural networks (CNNs), are widely used in healthcare for tasks like medical image recognition. These models can analyze complex medical images, such as MRIs or X-rays, and identify patterns associated with various conditions, enabling early detection of diseases like cancer. The ability of deep learning models to automatically learn hierarchical features from images has significantly improved diagnostic accuracy and efficiency in medical imaging. - Natural Language Processing in Virtual Assistants:
Application: Conversational AI
Description: Virtual assistants like Siri, Google Assistant, and Amazon Alexa leverage Complex AI models, including recurrent neural networks (RNNs) and transformer models like BERT (Bidirectional Encoder Representations from Transformers). These models enable natural language understanding, allowing virtual assistants to comprehend and respond to user queries in a conversational manner. They can perform tasks such as answering questions, setting reminders, and even engaging in context-aware dialogues, showcasing the complexity and versatility of these AI models.
These examples highlight how Complex AI models excel in handling sophisticated tasks, ranging from intricate image analysis in healthcare to the nuanced understanding of natural language in conversational AI systems. Their ability to learn complex representations from data has led to significant advancements in various domains.
AI in Relation to Problem-Solving Approaches
In dissecting the multifaceted world of AI, this section probes into the diverse problem-solving approaches it employs. From the significance of Rule-Based Systems in decision-making to the pivotal role of Search Algorithms and the transformative impact of optimization, we unravel the intricacies of AI’s strategic problem-solving capabilities.
Rule-Based Systems
Rule-based systems significantly contribute to AI problem-solving, offering a structured approach to decision-making. Industries such as finance, where rule-based algorithms automate trading strategies, highlight the success of this approach.
Expert perspectives and statistics underscore the reliability of Rule-Based Systems, making them a preferred choice in scenarios where adherence to predefined rules is essential.
Search Algorithms
Pivotal in AI problem-solving approaches, Search Algorithms enhance decision-making processes by systematically exploring possibilities. These algorithms play a central role, from route optimization in logistics to information retrieval in search engines.
Real-world applications include route planning and navigation systems, machine learning model optimization, engineering design and optimization, combinatorial optimization problems, and game AI and decision-making.
Optimization in AI
Fitting into the spectrum of AI problem-solving strategies, Optimization focuses on refining processes and maximizing outcomes. Optimism-centric approaches contribute to efficiency and cost-effectiveness, from supply chain management to resource allocation.
Navigating trade-offs inherent in optimization-centric approaches requires a nuanced understanding of specific industry requirements and constraints.
Future Trends and Emerging Types of AI
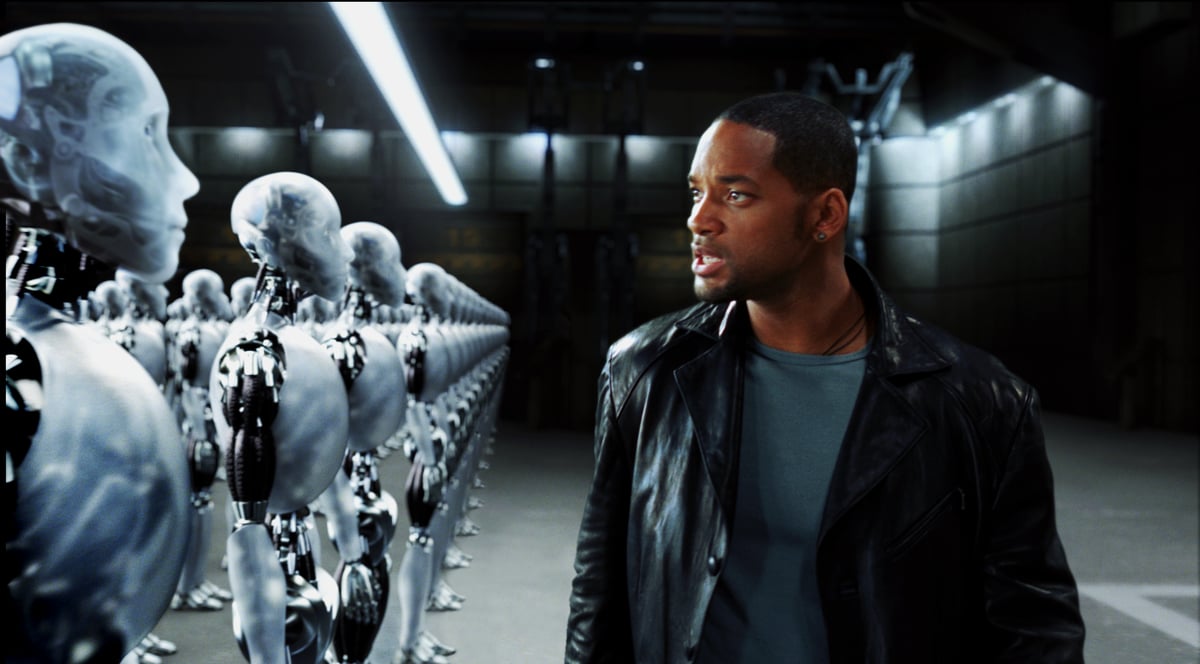
Embarking on a journey into the future of artificial intelligence, this section anticipates transformative trends and emerging AI types. From the potential redefinition brought by Quantum AI to the ethical considerations surrounding Sentient AI and the collaborative revolution heralded by Swarm Intelligence, we explore the cutting-edge of AI evolution.
Quantum AI
The future of AI holds promise with emerging technologies, and Quantum AI stands at the forefront. Redefining the AI landscape, Quantum AI leverages quantum computing principles to perform computations at speeds unattainable by classical computers.
Statistics indicate the potential for exponential speedup in solving complex problems. Expert opinions foresee Quantum AI revolutionizing industries that demand unprecedented computational power.
Sentient AI
Sentient AI refers to artificial intelligence systems designed with the capability to perceive, understand, and respond to their environment or users in a manner that emulates human-like intelligence. These systems often incorporate advanced techniques such as machine learning, natural language processing, and computer vision to enable contextual awareness and adaptive behavior. The goal of Sentient AI is to create machines that can demonstrate a level of consciousness, learning, and decision-making akin to human intelligence.
As AI evolves, ethical considerations become paramount, especially with the development of Sentient AI. Endowed with human-like cognitive abilities, Sentient AI raises questions about privacy, accountability, and the ethical deployment of intelligent systems.
In the movie The Matrix, AI systems achieve sentience and create a simulated reality (the Matrix) to control and manipulate human minds. The narrative explores themes of control, reality, and the potential dangers of unchecked AI development. While it can be entertaining for science fiction enthusiasts, it emphasizes the potential risks associated with the emergence of highly advanced and self-aware artificial intelligence.
Safeguards for responsible deployment are imperative, with experts emphasizing the need for transparent and accountable frameworks to ensure the ethical development of Sentient AI.
Swarm Intelligence
Another transformative trend on the horizon is Swarm Intelligence, drawing inspiration from collective behavior observed in natural systems. This approach involves coordinating a large number of simple AI agents to solve complex problems collaboratively.
Real-world applications range from traffic management to disaster response, showcasing the potential of Swarm Intelligence in revolutionizing collaborative problem-solving on a global scale.
Choosing the Right AI Solution
Since the world of AI presents enterprises with endless possibilities, selecting the right solution is a critical decision for decision-makers. From the fundamental distinctions between Narrow AI and General AI to the emerging frontiers of Quantum AI and Sentient AI, the journey into the world of AI is one of continuous discovery.
Understanding the practical applications, complexities, and future trends empowers enterprises to make informed decisions. As the AI landscape evolves, the role of trusted AI partners becomes increasingly crucial. Embracing AI with confidence and leveraging it as a strategic asset positions organizations for success in the ever-evolving digital era.
Core expertise and experience, makes Iterators the premier partner for organizations navigating the complexities of AI implementation. We’re happy to have you talk to us immediately to help you build critical artificial intelligence solutions.